In statistics, data measurement scales can be divided into four types: nominal, ordinal, interval, and ratio scales. Understanding the differences among these four measurement scales is crucial for researchers to grasp. This is because the choice of data analysis in research is heavily influenced by the measurement scale of the variables, whether they are nominal, ordinal, interval, or ratio scales.
Statistics can also be divided into non-parametric and parametric statistics. Non-parametric statistics can be applied to variables measured using nominal and ordinal scales. On the other hand, parametric statistics can be used to analyze variables measured using interval and ratio scales.
For each analysis option, there are assumptions that need to be met to ensure consistent and unbiased estimation results. Therefore, it is essential for researchers to select the appropriate analysis based on the measurement scale of their data variables. Considering the importance of this matter, in this discussion, we will explore the differences between nominal, ordinal, interval, and ratio scales along with examples.
Nominal Data Scale
The nominal data scale is the lowest level among the existing data scales. In the nominal data scale, the measured variables are only intended for differentiation purposes. In this case, variables measured with a nominal scale do not indicate any levels or hierarchy. Instead, variables are measured by categorizing them with the aim of distinguishing between categories.
Examples of nominal data scales include gender, occupation, and others. Let’s examine each example, such as the gender variable and occupation variable. In the gender variable, categorization is done to differentiate between male and female. This variable does not indicate any level that suggests one gender is higher or lower than the other.
To further illustrate, let’s consider the occupation variable. Occupations can include farmers, government employees, private sector employees, entrepreneurs, or others. In this case as well, the different occupations do not indicate any level that suggests one occupation is considered higher or better than others. Therefore, both the gender variable and occupation variable possess the characteristics of a nominal data measurement scale.
In the given examples, categorization is carried out purely for differentiation purposes without any levels. Data analysis for variables measured using a nominal scale can be conducted using non-parametric statistics. Non-parametric statistics generally have less stringent assumptions compared to parametric statistics.
Ordinal Data Scale
The next data measurement scale is the ordinal data scale. Variables measured using the ordinal data scale still under non-parametric statistics. Variables measured using this scale possess the characteristics of nominal data scale, but the difference lies in the presence of levels or ranks.
Hence, the key distinguishing factor between nominal and ordinal data scales is that while the nominal scale merely distinguishes between categories, the ordinal scale not only differentiates but also incorporates levels or ranks. Examples of ordinal data scales include educational level, behavior, motivation, preferences (variables measured using the Likert scale), and other variables that indicate levels or ranks.
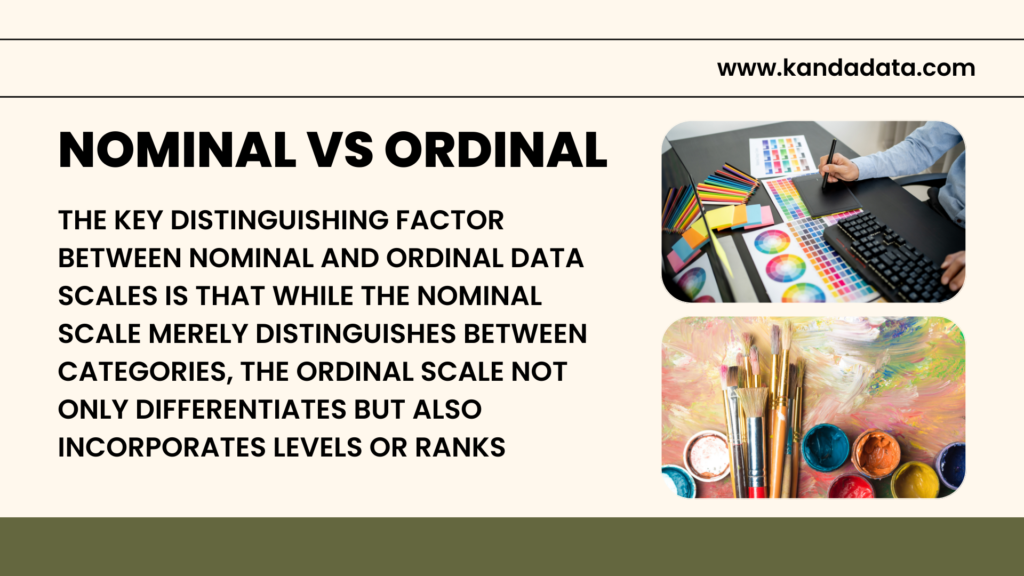
Based on the example of the educational level variable, we can observe that education can consist of primary school, junior high school, senior high school, and college. The answer choices for educational level not only differentiate between different levels of education but also indicate levels or ranks.
Based on this example, we can confidently state that Senior High School education is higher than Junior High School education. Additionally, it can be affirmed that individuals with college education are higher in terms of educational attainment compared to those with primary school, junior high school, or senior high school education.
Furthermore, variables measured using the Likert scale, such as behavior, motivation, consumer preferences, and competencies, are also measured using the ordinal scale. This is because measuring these variables requires statement items that represent the variable being measured, with response options ranging from strongly agree, agree, neutral, disagree, to strongly disagree.
Based on the statements in the Likert scale, it is evident that there are levels. If the variable being measured aims to assess agreement with a certain condition, then the response option of strongly agree would be preferable compared to disagree. Additionally, for the categorization of other variables where each response option of a variable indicates a level, it also falls within the ordinal scale.
Interval Data Scale
The next measurement scale is the interval scale, which falls under parametric statistics. Distinguishing variables measured using the interval scale from those measured using the nominal and ordinal scales is quite straightforward. Variables measured using the interval scale directly obtain numeric values.
For example, when someone observes the temperature in different geographical regions. Based on the measurement results, the researcher would obtain numerical values representing the temperature. In contrast, variables such as behavior, motivation, and occupation require scoring techniques to be transformed into numerical values.
So, variables measured using the interval scale are variables that directly obtain numerical values, but within them, there is a distance or interval and they do not indicate an absolute zero value.
To further facilitate understanding, another example of an interval variable is student learning outcomes. In student learning outcomes, there are intervals, such as the lowest student score being 0 and the highest student score being 100. From this example of student scores, it is known that the data directly obtains numerical values, has an interval, and does not have an absolute zero.

Variables that do not have an absolute zero cannot undergo multiplication operations. For example, let’s consider a student with a score of 90, which is considered three times smarter than a student with a score of 60. If we perform the mathematical operation of multiplying 60 by 3, the result would be 180. However, the maximum score for a student is 100. Therefore, in numerical values that have intervals, an absolute zero does not exist.
Ratio Data Scale
The ratio data scale is the highest level of measurement compared to other scales. The ratio data scale possesses all the characteristics of nominal, ordinal, and interval measurement scales, but the key difference is that it has an absolute zero value. The ratio data scale is also included in parametric statistics.
Examples of ratio data scale include profit data, input quantity, fertilizer quantity, seed quantity, production, demand, and so on. Based on the examples, the profit variable data shows that in profit measurement, it already exhibits an absolute zero value and can directly obtain numerical values. With profit data, we can perform multiplication operations, for instance, if the profit of business A is twice as high as the profit obtained by business B.
Generally, many variables used for data analysis in parametric statistics utilize the ratio data scale. However, when choosing to conduct analysis using parametric statistics, attention must be paid to the assumptions that need to be fulfilled to ensure consistent and unbiased estimation results.
Conclusion
Based on the information presented in the previous paragraphs, it is time to draw a conclusion. Based on the measurement scale, data can be divided into nominal, ordinal, interval, and ratio scales. Nominal and ordinal scales fall under nonparametric statistics, while interval and ratio scales fall under parametric statistics.
Researchers need to carefully consider the measurement scale of the variables used when selecting the appropriate statistical analysis tool. Each choice of data analysis has prerequisite assumptions that need to be met to ensure unbiased and consistent estimation results. Thus, the conclusion of research findings can be scientifically justified. This concludes the article I have written, hoping it is beneficial and provides additional knowledge for all of us. Stay tuned for the next article update from Kanda Data. Thank you.