Once the researcher has successfully conducted linear regression analysis, the next step is to interpret the results. It is crucial for the researcher to possess sufficient knowledge to interpret the findings. The interpretation based on these results can be used to draw conclusions from the research.
To begin discussing the interpretation of linear regression analysis output, it is necessary to have an understanding of relevant theories and previous research findings. This is important because when researchers interpret their results, they need to compare them with existing theories or previous research findings.
However, it is important for the researcher to ensure that all required assumptions are met. Specifically, for linear regression analysis using the Ordinary Least Squares (OLS) method, the researcher needs to check for the assumption prerequisites. This is done to generate consistent and unbiased estimations.
When interpreting the results of linear regression analysis, there are at least three key aspects that need to be interpreted and discussed. These aspects include the coefficient of determination (R squared), the F-statistic, and the t-statistic. Let us discuss the interpretation of each of these aspects in more detail one by one.
R squared
R squared, or the coefficient of determination, indicates how well the independent variables explain the dependent variable. The value of the coefficient of determination ranges from 0 to 1. The coefficient of determination can also be used to determine the goodness of fit of a model.
In a regression model, a higher value of the coefficient of determination indicates a better model. This is because the researcher has successfully specified the equation with independent variables that influence the dependent variable.
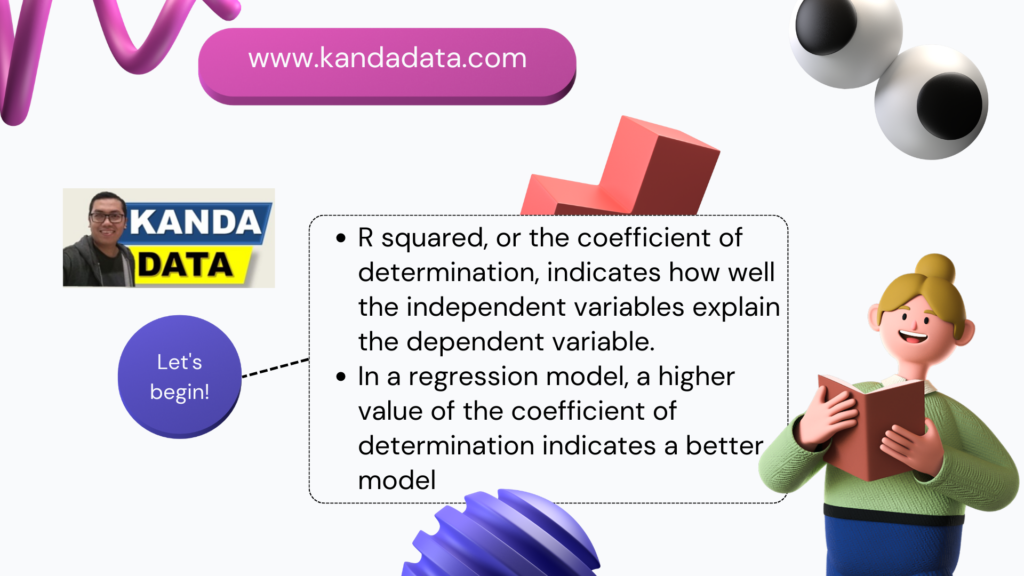
To interpret the coefficient of determination, let’s say a regression equation yields a coefficient of determination of 0.85 based on the test results. This coefficient of determination can be interpreted as 85% of the variation in the dependent variable can be explained by the variation in the independent variable. The remaining 15% is explained by other independent variables not included in the regression model.
F-Statistics
The next step in the interpretation process involves examining the Anova table in the linear regression output. The Anova table consists of calculations that lead to the F-statistic value. The F-statistic value can be used to test the hypothesis of simultaneous influence of independent variables on the dependent variable.
For example, let’s consider a research hypothesis that household income and expenditure have a significant impact on meat consumption. If the test results yield an F-statistic value of 30 and a p-value of 0.0012, the researcher can test the hypothesis using two criteria.
The first criterion is comparing the F-statistic value with the critical F-value from the F-table. If the F-statistic is greater than the critical F-value, the null hypothesis is rejected.
The second criterion is examining the probability of error (alpha). If the p-value is smaller than 0.05 (alpha), the null hypothesis is rejected.
After comparing the F-statistic with the critical F-value or examining the p-value, it can be concluded that the null hypothesis is rejected. Since the null hypothesis is rejected, the alternative hypothesis is accepted. Thus, it can be interpreted that household income and expenditure have a significant impact on meat consumption.
T-Statistics
T-statistics can be used to test the hypothesis of partial influence of independent variables on each dependent variable. In principle, the testing of t-statistics can also use both criteria. The first criterion involves comparing the t-statistic value with the critical T-value from the T-table. The second criterion involves examining the probability of error (alpha) for each independent variable.
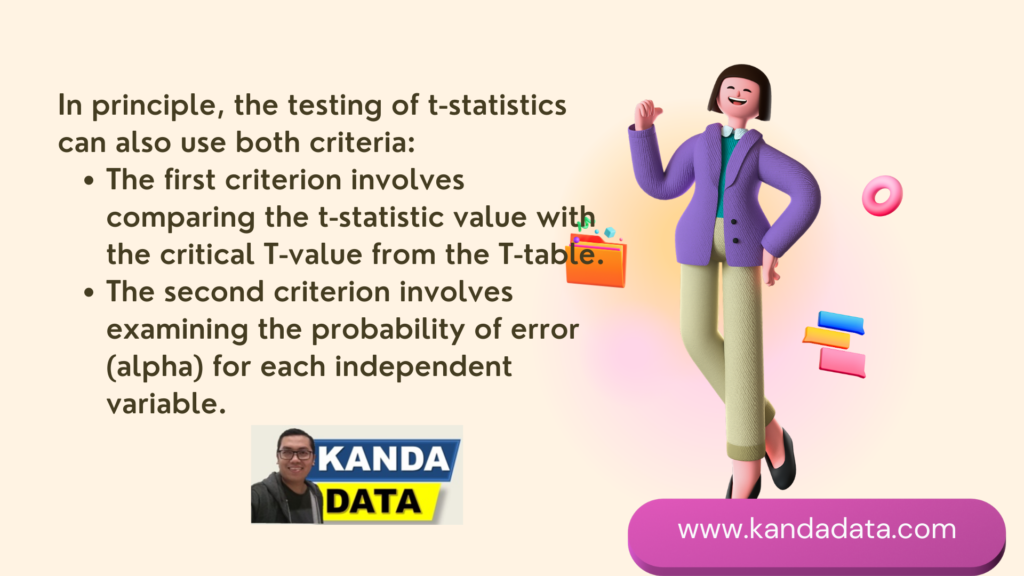
For example, let’s consider the coefficient value of the income variable as 2.4, with a t-statistic of 9.5 and a probability of error (p-value) alpha of 0.015. By using the criterion where the p-value < 0.05, the null hypothesis is rejected. Since the null hypothesis is rejected, the alternative hypothesis is accepted.
Thus, it can be interpreted that income partially has a significant effect on meat consumption. The positive value of the coefficient indicates that an increase in income is estimated to increase meat consumption.
Moving on to the coefficient value of the household expenditure variable, which is -1.6, with a t-statistic of -5.3 and a probability of error (p-value) alpha of 0.042. By using the criterion where the p-value < 0.05, the null hypothesis is rejected. Since the null hypothesis is rejected, the alternative hypothesis is accepted.
Thus, it can be interpreted that household expenditure partially has a significant effect on meat consumption. The negative value of the coefficient suggests that an increase in household expenditure is estimated to decrease meat consumption.
Conclusion
Based on the linear regression analysis output, researchers can interpret the coefficient of determination, F-statistic, and t-statistic. A coefficient of determination value approaching 1 indicates a better model.
The hypothesis testing for the simultaneous influence of independent variables on the dependent variable can be performed using the F-statistic. Additionally, the partial influence of independent variables on the dependent variable can be assessed using the t-statistic.
Well, this is the article that Kanda Data can write on this occasion. Hopefully, it provides benefits and new insights for all of us. Stay tuned for more articles from Kanda Data in the following week. Thank you.